Your Intuition Is a Statistical Genius — But Only if You Feed It the Right Data
- Ilana Bensimon
- Mar 25
- 21 min read
Updated: Mar 28
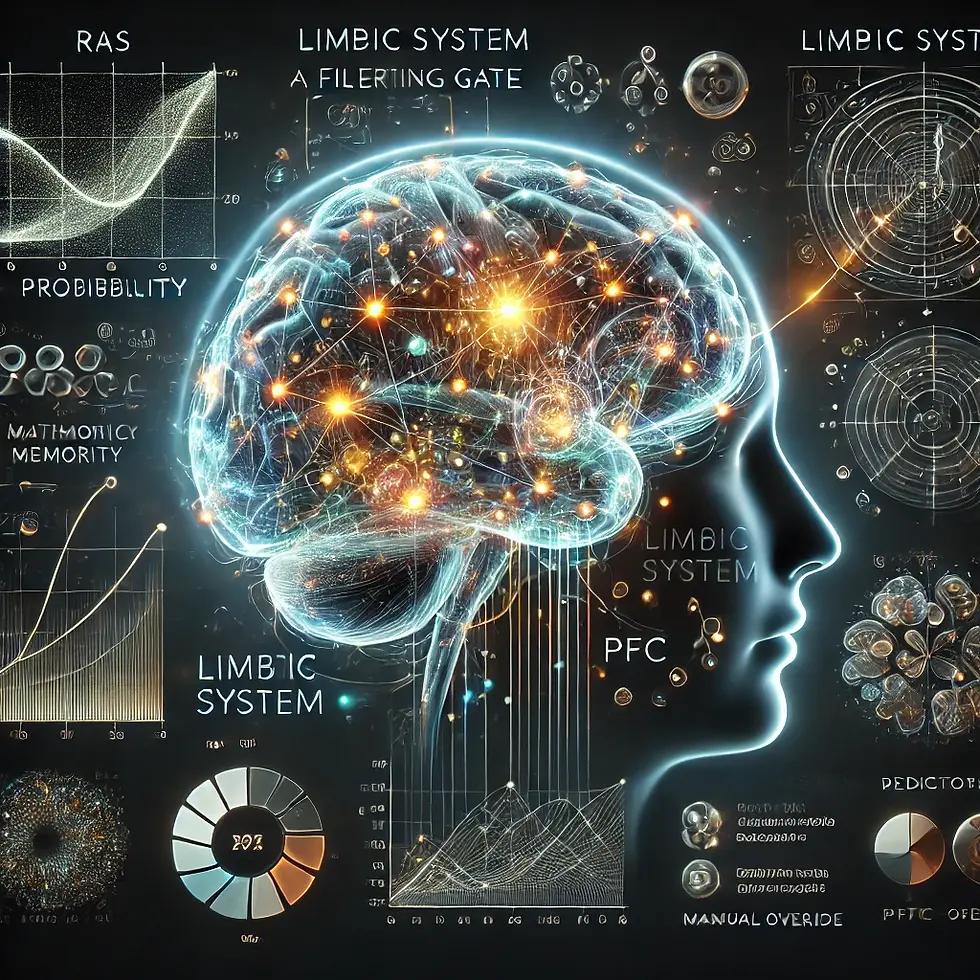
We tend to think of intuition as mystical — a gut feeling, a whisper from the universe.
But in reality? It’s statistical. Your brain is a high-powered but totally unconscious probability engine.
Every second, it’s scanning your environment, predicting what will be safe, what will be risky, and what will be rewarding — based on past experience.
It does all this in service of one goal:
To meet your basic and emotional needs with the least possible energy.
This is what nature optimizes for. You do it. Animals do it. And they do it well.
🦁 Lions predict prey movement based on time of day and past patterns.
🐘 Elephants remember where water is likely to be found across vast distances and shifting seasons.
That’s not magic. That’s pattern recognition. It’s Bayesian reasoning without a spreadsheet. It’s evolutionary game theory — running in the background of instinct.
And humans do the same. The difference?
Our system is more sophisticated — but our data is often corrupted. And even the best model fails when it’s built on flawed or outdated data.
So when you keep dating the wrong people, struggle with unnecessary anxiety, or sabotage your own success, the problem isn't your processing power. It's the quality of information your system is using to make predictions. Your internal statistician is working perfectly—it's just using bad data.
This article explores how to clean that data, update your models, and align your intuitive guidance system with your actual needs and goals in today's world.
No mysticism required—just a fascinating look at the biological mechanisms that drive our decisions and how to optimize them.
Animal Game Theorists: Instinct as Statistical Analysis
Humans aren't the only statistical analysts in the animal kingdom.
Consider these examples of evolved game theory:
The Stotting Gazelle: When spotted by a predator, gazelles often perform stiff-legged vertical jumps that seem counterproductive—they waste energy and delay escape. But these jumps actually function as statistical warnings, communicating: "I'm so fit I can waste energy on jumping; your probability of catching me is low." Research shows predators are less likely to chase gazelles that stot—they're reading the statistical signal.
The Strategic Cleaner Fish: Cleaner wrasses provide a service by removing parasites from larger fish that could easily eat them. Studies show these tiny fish behave more honestly (eating parasites rather than healthy tissue) when other potential client fish are watching. They're intuitively calculating reputation effects on their future feeding opportunities—a sophisticated cost-benefit analysis running on neural hardware.
These behaviors show how statistical reasoning is embedded in neural structures through evolution, creating intuitive behaviors that don't require conscious calculation.
Your Inner Prediction System, Simplified
Your intuition isn't a single capacity but emerges from three distinct neural systems that evolved with the same goal : to keep you alive in environments very different from today's world.
1. The Data Filter: Your Reticular Activating System
Imagine having to consciously process every sensory input hitting your nervous system—the pressure of your clothes against your skin, the ambient temperature, distant traffic sounds, the feeling of your tongue in your mouth. You'd be overwhelmed in seconds.
Your Reticular Activating System (RAS) prevents this by filtering millions of bits of sensory data down to a manageable 40-50 bits that reach conscious awareness. It's the evolutionary solution to deal with information overload.
The RAS decides what gets through based on:
What posed survival threats to your ancestors (sudden movements, unfamiliar sounds)
What you've programmed it to value (your name in conversation, your child's cry)
What doesn't match your predictions (unexpected outcomes)
This filtering happens before conscious thought—which means your RAS determines what data your brain uses for its calculations before you're even aware of making a decision.
2. The Pattern Library: Your Limbic System
Once data passes through your filter, it reaches your limbic system—the emotional core of your brain that's been evolving for over 150 million years. This part stores memories and builds unconscious models of how the world works. It doesn’t prioritize truth — it prioritizes predictability and past survival patterns. It’s fast and automatic. But not always relevant to your current reality.
This region contains:
The Amygdala: Tags experiences with emotional significance, ensuring you remember what helped or harmed you. This is why emotionally charged memories remain so vivid—your brain flagged them as survival-relevant information.
The Hippocampus: Organizes experiences into accessible patterns, connecting new information with existing models. When you "just know" something without knowing how you know it, your hippocampus is retrieving pattern matches too quickly for conscious awareness.
The Anterior Cingulate Cortex: Detects mismatches between expected and actual outcomes, flagging prediction errors that might require updating your models. That uneasy feeling when something's "off" often originates here.
3. The Executive Override: Your Prefrontal Cortex
The newest addition to your neural architecture is your prefrontal cortex—the region responsible for planning, analysis, and inhibiting impulses. It's the only system capable of questioning the output from your limbic system and consciously updating your predictive models.
This ability to override automatic responses is what separates human intelligence from other species. But there's a catch: your prefrontal cortex is energy-intensive and slow compared to your rapid, efficient limbic system.
This explains why intuitive responses often override rational analysis in moments of stress or fatigue—your brain defaults to its energy-efficient systems when resources are limited.
Why Your Brain Runs Probabilities
Our human brain consumes 20-25% of our body's energy budget while constituting just 2% of our body mass. This metabolic demand created intense evolutionary pressure to minimize energy expenditure through predictive processing.
Rather than reactively responding to changes (which would require constant energy output), your neural systems continuously generate statistical predictions to:
Maintain Homeostasis: Your brain constantly forecasts whether your internal parameters (blood sugar, temperature, hydration) will remain within optimal ranges, initiating behaviors to address predicted imbalances before they become problematic.
That afternoon craving for your desk drawer snack? It's your brain predicting a blood sugar drop before it happens. The thirst during exercise isn't current dehydration—it's forecasting future needs. These mechanisms evolved because anticipating resource needs before reaching critical levels provided massive survival advantages.
Optimize Social Standing: As deeply social animals, humans evolved sophisticated systems for predicting how their behaviors will affect group acceptance—a critical survival factor for our ancestors.
The way you instinctively lower your voice for sensitive topics, mentally rehearse difficult conversations, or feel that flash of embarrassment remembering past social missteps—all are your social prediction system at work. Even unconsciously matching others' speech patterns and body language represents automated social cohesion programming. These calculations mattered enormously when social rejection could mean death for our ancestors.
Maximize Resource Efficiency: Your brain constantly calculates effort-to-reward ratios, steering you toward high-yield, low-energy activities when possible.
Taking the elevator without conscious deliberation, feeling satisfaction at finding a shorter route, or experiencing reluctance to start an overwhelming project all reflect your brain's effort-to-reward calculations. Even procrastination often represents your system's prediction that the task will require less energy later. These efficiency mechanisms evolved because ancestors who conserved energy for critical survival activities outlived and outreproduced those who didn't.
These predictions operate as probability distributions rather than binary calculations. When you feel drawn toward or away from a situation, you're experiencing the output of these sophisticated statistical models.
Example:
"If I express vulnerability, what’s the probability I’ll be rejected?"
"If I take a break now, how likely am I to fall behind?"
"If I pursue this idea, will it pay off — or drain me?"
You don't consciously calculate those. But your nervous system does — based on the data you’ve fed it over time.
So here’s the critical question:
Are your internal models accurate? Or are they still running on biased inputs, fear-based assumptions, and outdated beliefs?
How Did Your Data Get Corrupted?
Even the most powerful statistical engine fails with corrupted data. Your neural prediction system suffers from the same statistical biases that plague data science—but with biological roots:
Developmental Sampling Bias
Just as statisticians worry about unrepresentative samples skewing results, your brain's predictive models get skewed by early experiences:
Small Sample Size Error: This error occurs when your brain draws sweeping conclusions from too few experiences. Childhood provides a limited dataset, yet your neural prediction system uses these few data points to form lifelong patterns.
Imagine a child who experiences rejection from peers three times during early school years. For the developing brain, these three incidents might represent nearly all their recorded social data. Their developing brain doesn't think, "This is an insufficient sample size"—it does what it evolved to do: form a working model from available information. The neural prediction system forms a sweeping conclusion: "social situations lead to rejection."
This happens because early humans faced life-or-death learning scenarios where waiting for large datasets was impossible. A child couldn't afford to encounter a predator twenty times before recognizing the danger pattern. Your brain evolved to extract maximum predictive value from minimal examples—prioritizing quick learning over statistical accuracy.
The problem persists because your brain doesn't automatically flag these conclusions as "preliminary findings based on insufficient data." Instead, it treats these patterns as established truths, despite the sample size being far too small for statistical reliability.
Temporal Data Weighting Error: This error concerns when you receive information, not how much. Your brain gives disproportionate weight to early experiences simply because they occurred during specific developmental windows.
Consider two identical rejection experiences—one at age 7 and one at age 27. Your neural architecture physically encodes the childhood experience more deeply because it happened when your brain was establishing its foundational wiring. Those early years feature heightened neuroplasticity—periods when connections form more readily and permanently.
Evolution favored this temporal bias because lessons learned in childhood needed to persist reliably into adulthood. Skills for finding food or avoiding dangers shouldn't be easily forgotten, so the brain developed "sensitive periods" when experiences write themselves more permanently into your neural circuitry.
The error occurs because your brain continues treating information from these early periods as inherently more authoritative than later evidence, regardless of which might be more accurate or relevant to your adult life.
Together, these two biaises create a powerful combination: your childhood experiences provide both too few examples (small sample) AND get encoded more permanently (temporal weighting), making your intuitive predictions particularly vulnerable to statistically unsound patterns formed during early life.
Another sampling biais well known in data science affects our neural prediction system:
Neurological Survivorship Bias: This manifests in two critical ways.
First, emotionally significant events receive preferential encoding—creating a dataset filled with "survivors" (memories with strong emotional tags) while routine experiences fade.
Second, and perhaps more limiting, your dataset only contains experiences you actually had—completely missing all potential outcomes from paths not taken. Like analyzing only the planes that returned from combat rather than those shot down, or studying only the businesses that succeeded while ignoring failed ones, your brain works with a fundamentally incomplete picture of reality. The paths you avoided due to fear or comfort-seeking never generate data points in your system, creating prediction models based exclusively on the subset of life possibilities you've already experienced.
What makes these errors even more persistent is how your protective behaviors create self-reinforcing feedback loops. When your brain predicts "social situations are threatening" based on childhood rejections, it generates avoidance behaviors—declining invitations, minimizing participation in groups, or maintaining emotional distance. These protective strategies prevent you from collecting new data that might contradict your original prediction.
This creates a statistical trap: your limited, early experiences generate protective behaviors that actively prevent counter-examples from entering your dataset. Your prediction system then interprets this absence of negative outcomes (which resulted from avoidance, not safety) as confirmation that the avoidance was necessary. The protective behavior itself becomes evidence supporting the original flawed prediction, creating a closed loop that can persist for decades without corrective data.
This explains why someone who experienced childhood rejection will maintain the prediction "people will abandon me". They will likely disregard the stable relationships in their life and often engage in protective behaviours that will lead to more rejection, confirming their initial model. Their neural statistics are working with a systematically skewed dataset.
Predictive Confirmation Bias
Your brain also introduces processing errors that perpetuate inaccurate models:
Attentional Filter Bias: Just as algorithms can be programmed to seek confirming rather than disconfirming evidence, your RAS preferentially focuses on information matching existing predictions while filtering contradictory data—creating a closed feedback loop where inaccurate models become self-reinforcing.
Artificial Boundary Constraints: When your predictive system accepts statements like "I'm not creative" as parameters rather than hypotheses, it stops exploring outside those boundaries—exactly like a statistical model constrained by artificially narrow parameters that prevent it from finding optimal solutions.
Missing Outlier Detection: Quality statistical analysis requires mechanisms to identify systematic errors, but without external calibration, your brain lacks reliable methods to flag when its predictions consistently miss the mark—especially in domains where feedback is subjective or delayed.
When statisticians work with data, they implement formal methods to detect when their models are failing—automatic systems that flag unusual patterns or consistent prediction errors. Your neural architecture, however, evolved without this crucial quality-control mechanism.
For immediate survival threats, feedback is clear and instantaneous—touch fire, feel pain, update model. But for complex social predictions ("people like me"), emotional predictions ("this will make me happy"), or long-term life choices ("this career path is right"), the feedback becomes murky and delayed.
This missing error detection happens for several biological reasons:
First, delayed consequences don't trigger the immediate neurochemical signals needed for model updates. Your dopaminergic prediction system evolved to detect unexpected outcomes that occur within seconds or minutes, not years.
Second, and connected to boundary constraints, emotional investment in existing beliefs activates protective responses against contradictory evidence. Your brain treats established prediction models as survival-relevant information worth defending. When confronted with evidence that a core belief might be wrong, your amygdala activates a threat response, your stress hormones elevate, and your prefrontal cortex's analytical capabilities diminish—precisely when you need them most.
Third, social validation often reinforces flawed models. When others share similar prediction errors (like cultural myths about success or happiness), your brain interprets this agreement as evidence that its models are correct.
From an evolutionary perspective, this makes sense. Our ancestors faced immediate survival challenges where prediction accuracy was directly tied to survival. They didn't need sophisticated error detection for long-term life satisfaction or complex social dynamics—they needed quick pattern recognition with high sensitivity to potential threats.
This explains why external calibration—therapy, mentorship, feedback from trusted others—becomes so valuable. These outside perspectives serve as the outlier detection mechanism your brain didn't evolve to include, helping identify systematic errors in your predictions that you're biologically predisposed to defend rather than correct.
Real life example: The Automatic Yes
Take Michael who says yes to every request, regardless of his own needs or schedule. This people-pleasing pattern emerged from a clear childhood statistical lesson: when he accommodated others' demands, he received approval and avoided tension; when he expressed his own needs, he often faced criticism or disappointment.
His brain's prediction system calculated a simple but powerful equation: "Saying yes = safety and connection; saying no = rejection and conflict."
What makes this pattern particularly revealing is how his body responds before conscious thought occurs. When someone makes a request, his automatic "yes" emerges before he's even processed what's being asked. Only afterward does he feel the familiar weight of overcommitment.
Though Michael consciously knows his friends and colleagues would respect his boundaries, his prediction system continues running calculations based on outdated childhood data. This creates a growing gap between his external behavior (constant accommodation) and internal experience (increasing resentment and exhaustion)—a gap that will persist until he updates his brain's statistical model with new evidence that setting boundaries can lead to healthier relationships rather than rejection.
How To Debug Your Inner System? (Yes, You Can)
If you're into systems thinking, you can think of personal development as model refinement. The following five-step approach creates a comprehensive system for cleaning your mental data. Starting with diagnosis, then improving data quality, recalibrating early influences, optimizing energy, and finally expanding your dataset, each strategy addresses a specific aspect of your prediction system while building on the previous steps.
Step 1: Run Your Neural Prediction Diagnostic
Before attempting to clean your mental data, you need to identify which predictions are causing problems. Your automatic behaviors and emotional responses provide the most reliable window into your brain's statistical models.
Identify your default expectations: what outcomes your brain seems to anticipate by default. Common prediction patterns include:
Do you automatically expect rejection in social situations?
Anticipate criticism when sharing ideas?
Prepare for scarcity despite evidence of abundance?
Does your prediction system regularly anticipate failure despite evidence of capability?
These default predictions reveal what your neural models are optimized to detect.
Notice protective behavioral patterns: Pay attention to avoidance behaviors, preemptive defenses, or self-sabotaging patterns. These protective responses are direct outputs of your prediction system—visible evidence of the invisible statistical calculations happening beneath awareness. When you consistently engage in protective behaviors (avoiding eye contact, over-preparing, declining opportunities, hedging commitments), it often signals that your prediction model is calibrated too negatively, overestimating threats based on limited or outdated data. These behaviors are essentially your brain saying, "I calculate a high probability of pain in this situation" even when objective evidence doesn't support this forecast. Notice also how your prediction system often operates in black-and-white terms, treating minor discomfort and serious pain as equivalent threats. This binary thinking—another evolutionary holdover—leads to avoiding potentially growth-producing experiences that might involve temporary discomfort but offer significant long-term benefits.
Recognize emotional intensity mismatches: When your emotional reaction seems disproportionate to current circumstances (intense anxiety before routine meetings, overwhelming disappointment at minor setbacks), your brain is likely applying statistical weights from early experiences to present situations.
Map relationship repetitions: If you encounter the same problems across different relationships, from romantic to professional relationships, your prediction system is likely creating self-fulfilling prophecies—expecting certain outcomes so consistently that you inadvertently create them.
When you notice yourself anxiously preparing for rejection before a social gathering, or mentally rehearsing defenses against criticism before sharing an idea, you're witnessing your prediction engine at work. These anticipatory responses offer direct evidence of the statistical models your brain is running in the background.
This diagnostic process engages your prefrontal cortex—your brain's newest evolutionary equipment—to examine outputs from older limbic and subcortical systems. By working backward from observable patterns to underlying predictions, you identify precisely which mental models require updating.
Try this: Think about the last time you avoided something important, or had an relatively intense reaction to a situation —what prediction was your brain making? What did you fear?
Step 2: Enhance Data Collection Quality
Your prediction engine operates on the data it receives through your sensory and interoceptive systems. Improving this input stream is essential before attempting to recalibrate existing models.
Nervous system regulation: Your brain's ability to process new information depends heavily on your autonomic nervous state. When your system is in sympathetic dominance (fight-flight-freeze), your amygdala takes control, shutting down the very brain regions needed for updating mental models. Regular practices that activate your parasympathetic "rest and digest" system create the neurobiological conditions where learning and model updating can occur.
From an evolutionary perspective, this makes perfect sense. Your ancestors couldn't afford to revise their mental models during moments of perceived danger—they needed immediate, automatic responses. Only during periods of safety could their brains integrate new information and update predictions. By deliberately creating this state of regulated calm, you're signaling to your prediction system that it's safe to incorporate new data.
Body-based awareness training: Your nervous system constantly generates vital information that typically remains below conscious threshold. When you develop interoceptive awareness—the ability to detect internal signals from your own body—you gain access to data your prediction system needs for accurate forecasting.
This works because body sensations often represent your brain's first-level prediction calculations. The knot in your stomach before a social event isn't random—it's your enteric nervous system (your "second brain") responding to limbic predictions. By noticing these sensations without immediately reacting, you create a small but crucial gap between prediction and response where new learning can occur.
Try this: Set a timer for three random moments today. When it goes off, scan your body from feet to head, noting sensations without judging them. What information were you missing?
Step 3: Recalibrate Early Data Weighting
Once you've identified problematic prediction patterns and improved your data collection, the next step is to address the disproportionate statistical weight your brain gives to early experiences.
Neural reconsolidation approaches: Techniques like EMDR (Eye Movement Desensitization and Reprocessing) work by activating memory networks containing early experiences while simultaneously introducing new processing elements. This creates a "reconsolidation window"—a brief period when established neural pathways become temporarily malleable. During this window, your brain can update emotional associations attached to early memories, reducing their statistical influence on current predictions.
Hypnotic reframing: Hypnosis temporarily modifies your brain's filtering system—specifically reducing activity in brain networks that normally maintain your established beliefs. This receptive state allows new perspectives to reach the limbic regions that house your prediction engine more directly. Modern neuroscience shows that hypnosis creates heightened theta wave activity similar to states when your brain naturally updates its models.
Psychedelic-assisted therapy: Emerging research shows that substances like psilocybin, when used in controlled therapeutic settings, can create a unique brain state where deeply encoded neural patterns become more accessible for revision. These compounds temporarily reduce activity in the brain's default mode network—the system that maintains your consistent sense of self and established beliefs. This creates a neuroplastic state where the statistical weights assigned to early experiences can be recalibrated, potentially allowing long-standing prediction patterns to be updated with new information. From a neural perspective, these therapies may work by temporarily relaxing the brain's protective mechanisms around core programming, creating a biological state where fundamental updating becomes possible.
Targeted autosuggestion: Consistent repetition of alternative perspectives creates competing neural pathways to counter early programming. When you repeatedly tell yourself "I'm worthy of love and respect because i am a good person" you're essentially feeding your model with new data points that will weight more and more with the repetition.
Memory contextualization: Deliberately reviewing early formative experiences with your adult perspective engages your fully developed prefrontal cortex to process childhood memories that formed when this brain region was immature. This creates new neural associations that help your brain properly categorize these experiences as "data from an undeveloped prediction system" rather than reliable statistical evidence. One practical way of doing it would be to write a transgenerational story of your family, which will help you see your childhood experiences not as isolated personal events, but as links in a longer chain of intergenerational patterns.
These approaches directly address the temporal data weighting error in your neural prediction system. By reducing the outsized influence of early experiences, they help your brain develop a more statistically sound model based on your complete life dataset rather than overweighting a small sample from childhood.
Try this: Identify one childhood conclusion that still affects you. Write it down, then beside it, list five pieces of contradictory evidence from your adult life.
Step 4: Optimize Your Energy Budget
Your brain's willingness to update its prediction models depends heavily on perceived energy availability. When your system detects energy scarcity, it automatically shifts toward conservative prediction strategies that rely on existing patterns rather than creating new ones.
Address physical energy drains: Your brain's disproportionate energy requirement means that physical depletion directly impacts cognitive flexibility. Unstable blood glucose, poor sleep quality, and chronic inflammation all trigger energy conservation modes in your brain, making it less willing to invest in the metabolically expensive process of updating neural networks.
From an evolutionary perspective, this makes perfect sense. When your ancestors faced food scarcity or physical threats, their brains needed to conserve energy for immediate survival rather than devoting precious glucose to revising mental models. In those environments, relying on established patterns was more energy-efficient than creating new neural pathways.
Try this: Track your prediction quality alongside physical markers like sleep quality, meal timing, and exercise. Most people discover clear patterns where physical depletion correlates with increased reliance on outdated mental models and reactive behaviors.
Reduce cognitive load: Your attentional systems have limited capacity, and overloading them creates a form of energy depletion. The constant alerts, decisions, and information processing demands of modern environments force your brain into energy conservation mode. When your cognitive resources are overtaxed, your prediction system automatically defaults to established patterns rather than incorporating new data.
Try this: Implement regular periods of focused monotasking and digital disconnection. Research shows that even brief attentional restoration significantly improves the neural resources available for updating prediction models.
Regular time in natural settings resets perceptual baselines. Modern environments bombard your system with novel stimuli densities your brain never evolved to process continuously. Nature provides the sensory distribution your neural architecture was designed for.
Transform inner dialogue: The language patterns you use in self-talk directly impact your brain's energy budget. Self-critical inner dialogue activates threat-detection circuits in your amygdala and anterior cingulate cortex, triggering stress responses that divert energy away from learning networks. Your brain processes harsh self-talk as a social threat, activating the same neural circuits that respond to external criticism.
When your inner voice constantly says things like "I always mess this up" or "I'll never be good enough," your brain responds by releasing cortisol and adrenaline—stress hormones that prepare you for threat but impair the neural networks needed for updating predictions. This creates a vicious cycle where energy depletion leads to more rigid thinking, which generates more problems, which triggers more self-criticism, creating further energy depletion.
Try this: Practice noticing absolutist language ("always," "never," "everyone," "no one") in your thought patterns. These terms trigger your brain's threat detection systems by framing situations in all-or-nothing terms that your ancestors would have associated with survival threats. Replace these energy-draining thought patterns with more nuanced language that your brain processes as less threatening.
Manage emotional energy leaks: Your limbic system doesn't distinguish between necessary and unnecessary emotional expenditures. Worrying about hypothetical futures, ruminating over past interactions, or engaging in social comparison all consume the same emotional energy resources that your brain needs for revising prediction models.
From an evolutionary perspective, your ancestors' environments contained a limited number of potential social and emotional challenges. Modern life presents your brain with virtually unlimited opportunities for emotional energy expenditure—many of which provide no adaptive advantage.
Try this: Conduct a weekly "energy audit" where you track activities, relationships, and thought patterns that deplete your emotional resources without contributing to your wellbeing. By consciously redirecting energy from these low-return investments, you create the surplus your brain needs to engage in the metabolically expensive process of updating its prediction models.
By systematically addressing these energy leaks, you create the biological conditions where your brain feels safe allocating resources to new learning rather than defaulting to established patterns. When your system detects energy abundance rather than scarcity, it becomes naturally more willing to invest in revising outdated predictions and collecting new data from previously avoided domains.
Try this: For one day, rate your energy level hourly (1-10). Note the activities, thoughts, or people present during significant drops.
Step 5: Expand Your Dataset Through Deliberate Exposure
After optimizing your neural energy budget, the next critical step is addressing the survivorship bias in your prediction system by deliberately collecting new data points from previously avoided domains.
Strategic comfort zone expansion: Your brain's prediction system suffers from a fundamental data problem—it only has information from experiences you've actually had. This creates prediction models based exclusively on a subset of life possibilities while missing data from paths not taken. From an evolutionary perspective, this data limitation made perfect sense. For your ancestors, venturing into unknown territory carried potentially fatal risks, so sticking with familiar patterns offered survival advantages.
In our modern environment, however, this same mechanism leads to unnecessarily restricted lives based on incomplete data. Your brain continues running statistical analyses that treat the absence of negative outcomes from avoided experiences as evidence that avoidance was necessary, rather than recognizing this as missing data.
Try this: Identify one domain where your prediction system consistently forecasts negative outcomes (social rejection, failure, criticism) and design a small experiment that challenges this prediction. Start with low-stakes situations where the potential downside is minimal but the information gained is valuable. Each new data point from previously avoided territory provides your brain with crucial evidence it needs to update its statistical models.
Diverse perspective exposure: Your prediction system evolved in an environment where most humans a person encountered shared similar beliefs and experiences. Today's world offers unprecedented access to diverse viewpoints, yet our brains naturally gravitate toward information that confirms existing models while filtering out contradictory data.
This filtering happens because your reticular activating system evolved to prioritize inputs that matched established neural patterns—an efficiency mechanism that prevented your ancestors' brains from wasting precious energy processing every available stimulus. In information-rich modern environments, however, this same mechanism creates echo chambers that reinforce potentially flawed predictions.
Try this: Deliberately seek perspectives that challenge your existing models, particularly in domains where your predictions have proven unreliable. This isn't about changing your mind, but about giving your brain's statistical engine access to more complete information.
Curiosity cultivation: Your brain's natural tendency toward confirmation bias—seeking evidence that supports existing beliefs—served an evolutionary purpose by creating stable prediction models. However, this same mechanism can prevent your neural systems from detecting and correcting statistical errors. Deliberately cultivating curiosity counteracts this tendency by activating reward circuits associated with information-seeking behavior.
When you approach situations with genuine curiosity rather than certainty, your brain releases dopamine and norepinephrine—neurochemicals that enhance neural plasticity and create the biological conditions for model updating. This happens because your distant ancestors who were curious about their environments discovered new resources and threats, creating survival advantages over those who relied exclusively on established knowledge.
Try this: Practice replacing evaluative thinking ("That's wrong") with curious thinking ("That's seems wrong to me—I wonder why?"). This simple language shift activates different neural networks, moving from your amygdala's threat-detection circuits to your prefrontal cortex's exploration systems. The phrase "I wonder..." particularly stimulates activity in brain regions associated with learning and memory consolidation, priming your neural architecture to incorporate new information rather than defending existing models.
By systematically expanding your dataset through these approaches, you address the fundamental limitation in your prediction system—its reliance on incomplete information. Each new experience from previously avoided domains provides critical evidence your brain needs to recalibrate its statistical models, gradually shifting your intuitive predictions to more accurately reflect reality rather than outdated patterns based on limited or early experiences
Try this: Identify one small action outside your comfort zone that you can take this week. Before doing it, write down what your brain predicts will happen. After doing it, compare your prediction with reality.
Conclusion: Intuition as Optimizable Biology
By treating intuition as a statistical prediction engine, we gain powerful tools for optimizing its function. The five strategies we've explored—diagnosing prediction patterns, enhancing data collection, recalibrating early weights, optimizing energy budgets, and expanding our datasets—work together to transform your internal guidance system from one calibrated for ancestral threats to one optimized for modern flourishing.
When your intuitive system runs on clean data:
You recognize genuine opportunities instead of defending against imaginary threats. Your brain evolved in environments where false negatives (missing a threat) were more costly than false positives (seeing threats that weren't there). This created a prediction system with a bias toward threat detection—a bias that served your ancestors well but can lead modern humans to miss opportunities while defending against low-probability dangers. Clean data recalibrates this bias, allowing your system to better distinguish between genuine risks and opportunities worth pursuing.
You allocate energy more efficiently. Your brain's prediction calculations consume significant metabolic resources. When these predictions repeatedly miss the mark, your system wastes energy preparing for outcomes that never arrive. By cleaning your mental data, you reduce these prediction errors, creating an energy surplus your brain can redirect toward growth, creativity, and connection rather than hypervigilance and defense.
You make decisions with less internal resistance. When your predictions align with reality, you experience what neuroscientists call "processing fluency"—the sensation of ease that comes when new information integrates smoothly with existing models. This fluency generates positive affect in your brain's reward centers, creating a sense of flow rather than friction as you navigate life choices.
You optimize not for protection—but for authenticity and meaning. Your ancient prediction system evolved primarily to keep you alive and included in your tribe. These evolutionary priorities created neural circuitry finely tuned for threat detection and social conformity. By updating this system with clean data, you recalibrate your internal compass toward what genuinely fulfills you rather than what merely protects you from harm or social rejection.
This is the ultimate purpose of cleaning your mental data: optimizing for inner coherence. When your predictions match your reality—when your internal statistical models accurately represent your environment and needs—you move through life with clarity, precision, and confidence. The emotional friction that comes from constantly recalibrating faulty predictions diminishes, creating a sense of alignment between your internal experience and external reality.
Intuition, then, becomes what it was designed to be: a fast, adaptive tool for navigating complexity—backed by a clear, clean internal model. Your gut feelings transform from potential sources of confusion or misdirection into reliable guides, their statistical calculations updated with relevant, properly weighted data rather than outdated patterns from early life.
Clean data. Clear signals. True navigation.
Comments